The power of biological replicates in statistical analysis
- Ghost
- Sep 19, 2020
- 2 min read
Updated: Mar 12
Biological replicates are commonly used in case-control studies for a fundamental reason: no two individuals are biologically identical. When investigating biological differences between individuals with distinct phenotypes, such as cancerous versus non-cancerous conditions, inherent biological variation within each phenotype—unrelated to the phenotype of interest—acts as a confounding factor. To mitigate this, an adequate number of samples must be collected from each phenotypic group to reduce the impact of such variation and reveal true biological differences. Additionally, while replicates are also essential for controlling technical variation, this aspect is beyond the scope of this article. Here, we will explore the influence of biological replicates on the selection of analytical approaches throughout the study
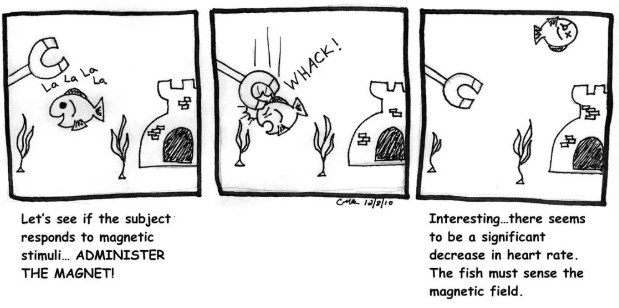
The Role of Biological Replicates in RNA-Seq and Differential Expression Analysis
One of the primary applications of biological replicates is in RNA-Seq, particularly in differential expression (DE) analysis. The negative binomial distribution is widely used to model gene expression counts while accounting for both technical and biological variation. Alternative methods, such as limma, utilize a normal distribution.
To understand the statistical basis of these models, we introduce the Central Limit Theorem (CLT), which states that when sample size is sufficiently large, the distribution of the test statistic approaches normality, regardless of the underlying distribution of the data. In DE analysis, the primary objective is to determine whether the average expression level of a gene differs significantly between conditions. Since the average expression level serves as the test statistic, it adheres to the CLT.
From this, we can draw the following conclusions:
When conducting an RNA-Seq experiment with a limited number of biological replicates, it is crucial to use a model that accurately captures biological variability. In DE analysis, this means relying on negative binomial-based models, as they best represent the biological scenario of interest.
When working with large publicly available datasets with abundant biological replicates (e.g., TCGA), simpler methods, such as Student’s t-test, can be effectively used without introducing significant bias. Similarly, in single-cell RNA-Seq (scRNA-Seq), where each cell represents a biological replicate, even more straightforward non-parametric methods, such as the Wilcoxon test, can be employed.
A similar principle applies to DNA methylation analysis. To identify Differentially Methylated CpG Sites (DMCs), traditional approaches often use Fisher’s exact test, which follows a hypergeometric distribution. However, this method has been challenged for not adequately accounting for biological variation—similar to the limitations of Poisson distribution-based DE analysis. As an alternative, negative binomial models have been proposed. However, when a study has access to a large number of biological replicates, this debate becomes less critical, as sufficient sample size can mitigate biological variability.
The Importance of Biological Replicates
The power of biological replicates lies in their ability to mitigate biological variation, a key confounder in case-control studies aimed at identifying biological markers. In general, the more biological replicates a study includes, the less it is affected by confounding factors, and the fewer constraints are imposed on the choice of statistical methods.